Deep Learning Model in Science Learning: Bibliometric Analysis
DOI:
https://doi.org/10.30736/seaj.v7i1.1160Keywords:
Deep Learning; Science Learning; Bibliometrics; EducationAbstract
The deep learning model is one of the learning models that can be applied in science learning. Research related to deep learning has grown very rapidly in recent years. Research on deep learning models has produced many theoretical and empirical findings. Many trends have emerged to highlight the complexity and dynamics of deep learning models in science learning. This study aims to discover the latest trends in deep learning model research in science learning. This study uses a bibliometric approach of analysis based on the Google Scholar database. Based on this study's title, abstract, and keywords, it produced 872 studies from 2015-2024. The results of this study show that the deep learning model in this learning has increased significantly in 2022. The increase in research on deep learning models shows the importance of applying deep learning models in learning.
Downloads
References
Agbo, F. J., Oyelere, S. S., Suhonen, J., & Tukiainen, M. (2021). Scientific production and thematic breakthroughs in smart learning environments: a bibliometric analysis. Smart Learning Environments, 8(1), 1–25. https://doi.org/10.1186/s40561-020-00145-4
Ajibade, S. S. M., Zaidi, A., Al Luhayb, A. S. M., Adediran, A. O., Voumik, L. C., & Rabbi, F. (2023). New Insights into the Emerging Trends Research of Machine and Deep Learning Applications in Energy Storage: A Bibliometric Analysis and Publication Trends. International Journal of Energy Economics and Policy, 13(5), 303–314. https://doi.org/10.32479/ijeep.14832
Ajibade, S. S. M., Zaidi, A., Bekun, F. V., Adediran, A. O., & Bassey, M. A. (2023). A research landscape bibliometric analysis on climate change for last decades: Evidence from applications of machine learning. Heliyon, 9(10), e20297. https://doi.org/10.1016/j.heliyon.2023.e20297
Ali, L., Alnajjar, F., Khan, W., Serhani, M. A., & Al Jassmi, H. (2022). Bibliometric Analysis and Review of Deep Learning-Based Crack Detection Literature Published between 2010 and 2022. Buildings, 12(4). https://doi.org/10.3390/buildings12040432
Amini, M., Sharifani, K., & Rahmani, A. (2023). Machine Learning Model Towards Evaluating Data Gathering Methods in Manufacturing and Mechanical Engineering. International Journal of Applied Science and Engineering Research, 15(4), 349–362. https://ssrn.com/abstract=4331917
Asnur, L., Jalinus, N., Faridah, A., Apra, T., Ambiyar, R. D., & Utami, F. (2024). Video-blogs ( Vlogs ) -based Project : A Meta Analysis. 14(5), 1553–1557.
Bhattacharya, S. (2019). Some salient aspects of machine learning research: A bibliometric analysis. Journal of Scientometric Research, 8(2), 85–92. https://doi.org/10.5530/jscires.8.2.26
Bidwell, R. V., Mishra, S., Patil, S., Shaw, K., Vora, D. R., Kotecha, K., & Zope, B. (2022). Deep Learning Approaches for Video Compression: A Bibliometric Analysis. Big Data and Cognitive Computing, 6(2). https://doi.org/10.3390/bdcc6020044
Chandra, A., Tünnermann, L., Löfstedt, T., & Gratz, R. (2023). Transformer-based deep learning for predicting protein properties in the life sciences. ELife, 12, 1–25. https://doi.org/10.7554/eLife.82819
Chen, X., Xie, H., Qin, S. J., Chai, Y., Tao, X., & Wang, F. L. (2024). Cognitive-Inspired Deep Learning Models for Aspect-Based Sentiment Analysis: A Retrospective Overview and Bibliometric Analysis. Cognitive Computation, 3518–3556. https://doi.org/10.1007/s12559-024-10331-y
Chen, X., Zou, D., Xie, H., & Wang, F. L. (2021). Past, present, and future of smart learning: a topic-based bibliometric analysis. International Journal of Educational Technology in Higher Education, 18(1). https://doi.org/10.1186/s41239-020-00239-6
Choudhary, K., DeCost, B., Chen, C., Jain, A., Tavazza, F., Cohn, R., Park, C. W., Choudhary, A., Agrawal, A., Billinge, S. J. L., Holm, E., Ong, S. P., & Wolverton, C. (2022). Recent advances and applications of deep learning methods in materials science. Npj Computational Materials, 8(1). https://doi.org/10.1038/s41524-022-00734-6
Dawana, I. R., Dwikoranto, Setiani, R., & Marsini. (2022). E-Book Learning Research in Physics Education during the Last Five Years: A Review and Bibliometric Study. Journal of Physics: Conference Series, 2392(1). https://doi.org/10.1088/1742-6596/2392/1/012016
Dewanto, D., Wantu, H. M., Dwihapsari, Y., Santosa, T. A., & Agustina, I. (2023). Effectiveness of The Internet of Things (IoT)-Based Jigsaw Learning Model on Students’ Creative Thinking Skills: A- Meta-Analysis. Jurnal Penelitian Pendidikan IPA, 9(10), 912–920. https://doi.org/10.29303/jppipa.v9i10.4964
Djeki, E., Dégila, J., Bondiombouy, C., & Alhassan, M. H. (2022). E-learning bibliometric analysis from 2015 to 2020. Journal of Computers in Education, 9(4), 727–754. https://doi.org/10.1007/s40692-021-00218-4
Edy Nurtamam, M., Apra Santosa, T., Aprilisia, S., Rahman, A., & Suharyat, Y. (2023). Meta-analysis: The Effectiveness of Iot-Based Flipped Learning to Improve Students’ Problem-Solving Abilities. Jurnal Edumaspul, 7(1), 2023–1492.
Ichsan, I., Suharyat, Y., Santosa, T. A., & Satria, E. (2023). Effectiveness of STEM-Based Learning in Teaching 21st Century Skills in Generation Z Student in Science Learning: A Meta-Analysis. Jurnal Penelitian Pendidikan IPA, 9(1), 150–166. https://doi.org/10.29303/jppipa.v9i1.2517
Ivanova, M., Grosseck, G., & Holotescu, C. (2024). Unveiling Insights: A Bibliometric Analysis of Artificial Intelligence in Teaching. Informatics, 11(1). https://doi.org/10.3390/informatics11010010
Jescovitch, L. N., Scott, E. E., Cerchiara, J. A., Merrill, J., Urban-Lurain, M., Doherty, J. H., & Haudek, K. C. (2021). Comparison of Machine Learning Performance Using Analytic and Holistic Coding Approaches Across Constructed Response Assessments Aligned to a Science Learning Progression. Journal of Science Education and Technology, 30(2), 150–167. https://doi.org/10.1007/s10956-020-09858-0
Khairi, S. S. M., Bakar, M. A. A., Alias, M. A., Bakar, S. A., Liong, C. Y., Rosli, N., & Farid, M. (2022). Deep Learning on Histopathology Images for Breast Cancer Classification: A Bibliometric Analysis. Healthcare (Switzerland), 10(1). https://doi.org/10.3390/healthcare10010010
Khashman, A., Sadikoglu, G., & Khashman, Z. (2020). Using Deep Learning as Prediction Model in Poll-Driven Social Science Applications. International Conference on …. https://doi.org/10.1007/978-3-030-64058-3_51
Li, L., Huang, X., & Liu, P. (2022). … Development of Science and Technology Finance and Logistics Industry in the Yangtze River Economic Belt: Coupling Analysis Based on Deep Learning Model. Computational Intelligence and …. https://doi.org/10.1155/2022/5185190
Li, Y., & Zhong, Z. (2024). Decoding the application of deep learning in neuroscience: a bibliometric analysis. Frontiers in Computational Neuroscience, 18(October), 1–9. https://doi.org/10.3389/fncom.2024.1402689
Luciana, O., Sjoraida, D. F., Santosa, T. A., Nugraha, A. R., & Zain, A. (2024). The Effect of Technology-Based Management Learning on Children's Organizational Skills Development : A Meta-Analysis Approach. Jurnal Obsesi: Jurnal Pendidikan Anak Usia Dini, 8(6), 1777–1786. https://doi.org/10.31004/obsesi.v8i6.6593
Miraftabzadeh, S. M., Martino, A. Di, Longo, M., & Zaninelli, D. (2024). Deep Learning in Power Systems: A Bibliometric Analysis and Future Trends. IEEE Access, 12(November), 163172–163196. https://doi.org/10.1109/ACCESS.2024.3491914
Mu, B., Chen, L., Yuan, S. J., & Qin, B. (2023). A radiative transfer deep learning model coupled into WRF with a generic Fortran torch adaptor. Frontiers in Earth Science, 11, 1149566.
Oktarina, K., Suhaimi, Santosa, T. A., Razak, A., Irdawati, Ahda, Y., Lufri, & Putri, D. H. (2021). Meta-Analysis: The Effectiveness of Using Blended Learning on Multiple Intelligences and Student Character Education During the Covid-19 Period. International Journal of Education and Curriculum Application, 4(3), 184–192. http://journal.ummat.ac.id/index.php/IJECA/article/view/5505
Ovcharenko, E., Koblov, D., & Gulenko, O. (2024). X-ray analysis of parameters of infraorbital foramen Bibliometric analysis of deep learning applications in dentistry Assessment of dental anxiety in children using different methods. International Dental Journal, 74, S216. https://doi.org/10.1016/j.identj.2024.07.044
Rustam, F., Khalid, M., Aslam, W., Rupapara, V., Mehmood, A., & Choi, G. S. (2021). A performance comparison of supervised machine learning models for COVID-19 tweets sentiment analysis. PLoS ONE, 16(2), 1–23. https://doi.org/10.1371/journal.pone.0245909
Santosa, T. A., & Aprilisia, S. (2022). Analisis Pendekatan Saintifik Dalam Pembelajaran Ipa Selama Pandemi Covid-19 Di Sekolah Dasar. Jurnal Didika : Wahana Ilmiah Pendidikan Dasar, 8(1), 92–101. https://doi.org/10.29408/didika.v8i1.5776
Saputra, N. A., Hamidah, I., & Setiawan, A. (2023). A Bibliometric Analysis of Deep Learning for Education Research. Journal of Engineering Science and Technology, 18(2), 1258–1276.
Septaria, K., Fatharani, A., Dewanti, B. A., & Najawa, W. C. (2024). Designing Pirposal-learning based Module integrated with Socio-Scientific Issue to Enhance Students’ Scientific Reasoning Skill. Indonesian Journal of Mathematics and Natural Science Education, 5(2), 122-134.
Irnananda, L., Septaria, K., & Susanti, I. (2024). Pengaruh Model Pembelajaran Pirposal Terhadap Science Attitude Siswa Sekolah Menengah Pertama Pada Pembelajaran IPA Topik Asam Basa. Jurnal Penelitian Sains dan Pendidikan (JPSP), 4(2), 117-133.
Vinasti, N. F., Dewanti, B. A., & Septaria, K. (2024). Empowering Middle School Science Student: Innovative Articulate Storyline Strategies for Enhancing Problem-Solving and Independent Learning. ICoCSE Proceedings, 1.
Septaria, K., & Rismayanti, R. (2022). The effect of scientific approach on Junior High school students’ Scientific Creativity and Cognitive Learning Outcomes. Jurnal Penelitian dan Pengkajian Ilmu Pendidikan: e-Saintika, 6(3), 173-189.
Septaria, K., & Fatharani, A. (2022). Manga versus webtoon: Alternative science learning module based on Dr Stone. Jurnal Inovasi Pendidikan IPA, 8(1), 11-22.
Septaria, K., Fatharani, A., & Yasa, A. D. (2022). COVID-19 is a Conspiracy Disease? Diagnostic Mental Models and Students' Cognitive Abilities. Jurnal Penelitian Dan Pengkajian Ilmu Pendidikan: E-Saintika, 6(1), 18-32.
Septaria, K., & Fatharani, A. (2022). Analisis Penggunaan Software PlantNet Terhadap Pemerolehan Konsep Dan Keterampilan Prosedural Siswa. Indonesian Journal of Educational Science (IJES), 5(1), 73-83.
Septaria, K. (2019). Developing Character Students Trough Spot Capturing Method in Science Education Learning. Science Education and Application Journal, 1(1), 39-45.
Shoaib, M., Shah, B., EI-Sappagh, S., Ali, A., Ullah, A., Alenezi, F., Gechev, T., Hussain, T., & Ali, F. (2023). An advanced deep learning models-based plant disease detection: A review of recent research. Frontiers in Plant Science, 14(March), 1–22. https://doi.org/10.3389/fpls.2023.1158933
Sobral, S. R. (2021). Two decades of research in e-learning: A deep bibliometric analysis. International Journal of Information and Education Technology, 11(9), 398–404. https://doi.org/10.18178/ijiet.2021.11.9.1541
Valencia-Arias, A., González-Ruiz, J. D., Verde Flores, L., Vega-Mori, L., Rodríguez-Correa, P., & Sánchez Santos, G. (2024). Machine Learning and Blockchain: A Bibliometric Study on Security and Privacy. Information (Switzerland), 15(1). https://doi.org/10.3390/info15010065
Wang, R., Huang, S., Wang, P., Shi, X., Li, S., Ye, Y., Zhang, W., Shi, L., Zhou, X., & Tang, X. (2024). Bibliometric analysis of the application of deep learning in cancer from 2015 to 2023. Cancer Imaging, 24(1), 1–17. https://doi.org/10.1186/s40644-024-00737-0
Wantu, H. M., Muis, A., Zain, A., Hiola, S. F., Agustina, I., Santosa, T. A., Yastanti, U., & Nugraha, A. R. (2024). Effectiveness of Think-Pair-Share and STEM Models on Critical Thinking in Early Childhood Education. Jurnal Obsesi: Jurnal Pendidikan Anak Usia Dini, 8(5), 1320–1330. https://doi.org/10.31004/obsesi.v8i5.6202
Wibawa, I. P. D., Kallista, M., & ... (2022). … Activity Recognition Using Deep Learning and Nonparametric Model with Some Exchanges in Karl Popper’s Viewpoint and Kuhn’s Paradigm in Philosophy of Science. JMECS (Journal of …. https://journals.telkomuniversity.ac.id/jmecs/article/view/2408
Wibawa, I. P. D., Kallista, M., & Phaijoo, G. R. (n.d.). Human Action Recognition Using Deep Learning and Nonparametric Model With Some Exchanges in Karl Popper’s Viewpoint and Kuhn’s Paradigm: A …. In academia.edu. https://www.academia.edu/download/102469281/2408-121-17384-5-10-20221111.pdf
Wissler, S., Rocca, J., & Kubitschek, D. (2005). D Eep I Mpact C Omet E Ncounter : Test, 1, 1–20.
Yang, Z., Yu, C. H., & Buehler, M. J. (2021). Deep learning model to predict complex stress and strain fields in hierarchical composites. Science Advances, 7(15), 1–10. https://doi.org/10.1126/SCIADV.ABD7416
Yuan, J., Liu, Y., & Zhao, F. (2017). Exploration and Analysis of Computer Science Teaching Model in Colleges Based on Deep Learning. pdf.hanspub.org. https://pdf.hanspub.org/ces20170200000_96769832.pdf
Zhong, S., Zhang, K., Bagheri, M., Burken, J. G., Gu, A., Li, B., Ma, X., Marrone, B. L., Ren, Z. J., Schrier, J., Shi, W., Tan, H., Wang, T., Wang, X., Wong, B. M., Xiao, X., Yu, X., Zhu, J. J., & Zhang, H. (2021). Machine Learning: New Ideas and Tools in Environmental Science and Engineering. Environmental Science and Technology, 55(19), 12741–12754. https://doi.org/10.1021/acs.est.1c01339
Zulkifli, Z., Satria, E., Supriyadi, A., & Santosa, T. A. (2022). Meta-analysis: The effectiveness of the integrated STEM technology pedagogical content knowledge learning model on the 21st-century skills of high school students in the science department. Psychology, Evaluation, and Technology in Educational Research, 5(1), 32–42. https://doi.org/10.33292/petier.v5i1.144
Zulyusri, Z., Santosa, T. A., Festiyed, F., Yerimadesi, Y., Yohandri, Y., Razak, A., & Sofianora, A. (2023). Effectiveness of STEM Learning Based on Design Thinking in Improving Critical Thinking Skills in Science Learning: A Meta-Analysis. Jurnal Penelitian Pendidikan IPA, 9(6), 112–119. https://doi.org/10.29303/jppipa.v9i6.3709
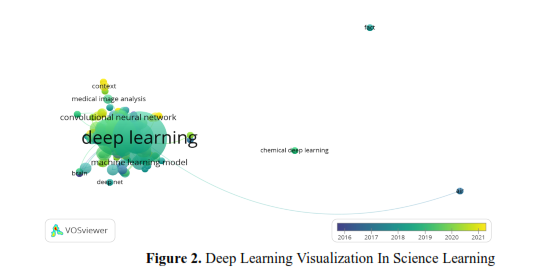
Downloads
Published
How to Cite
Issue
Section
License
Copyright (c) 2025 Nurina Happy, Tomi Apra Santosa, Siti Fatimah Hiola, Ifa Safira, Nur Latifah, Dewanto, Muh. Safar, Aat Ruchiat Nungraha

This work is licensed under a Creative Commons Attribution-ShareAlike 4.0 International License.
Authors who publish with this journal agree to the following terms:
- Authors retain copyright and grant the journal right of first publication with the work simultaneously licensed under a Creative Commons Attribution-ShareAlike 4.0 International License that allows others to share the work with an acknowledgment of the work's authorship and initial publication in this journal.
- Authors are able to enter into separate, additional contractual arrangements for the non-exclusive distribution of the journal's published version of the work (e.g., post it to an institutional repository or publish it in a book), with an acknowledgment of its initial publication in this journal.
- Authors are permitted and encouraged to post their work online (e.g., in institutional repositories or on their website) prior to and during the submission process, as it can lead to productive exchanges, as well as earlier and greater citation of published work (See The Effect of Open Access).

This work is licensed under a Creative Commons Attribution-ShareAlike 4.0 International License.